
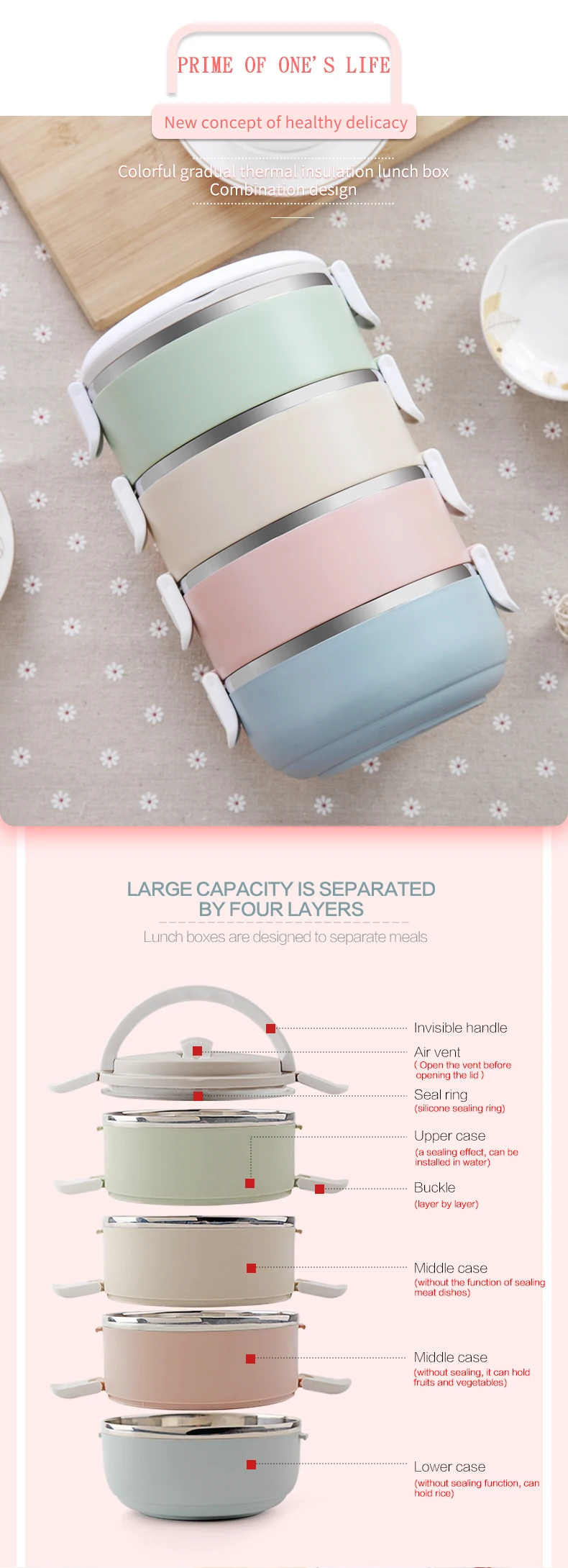
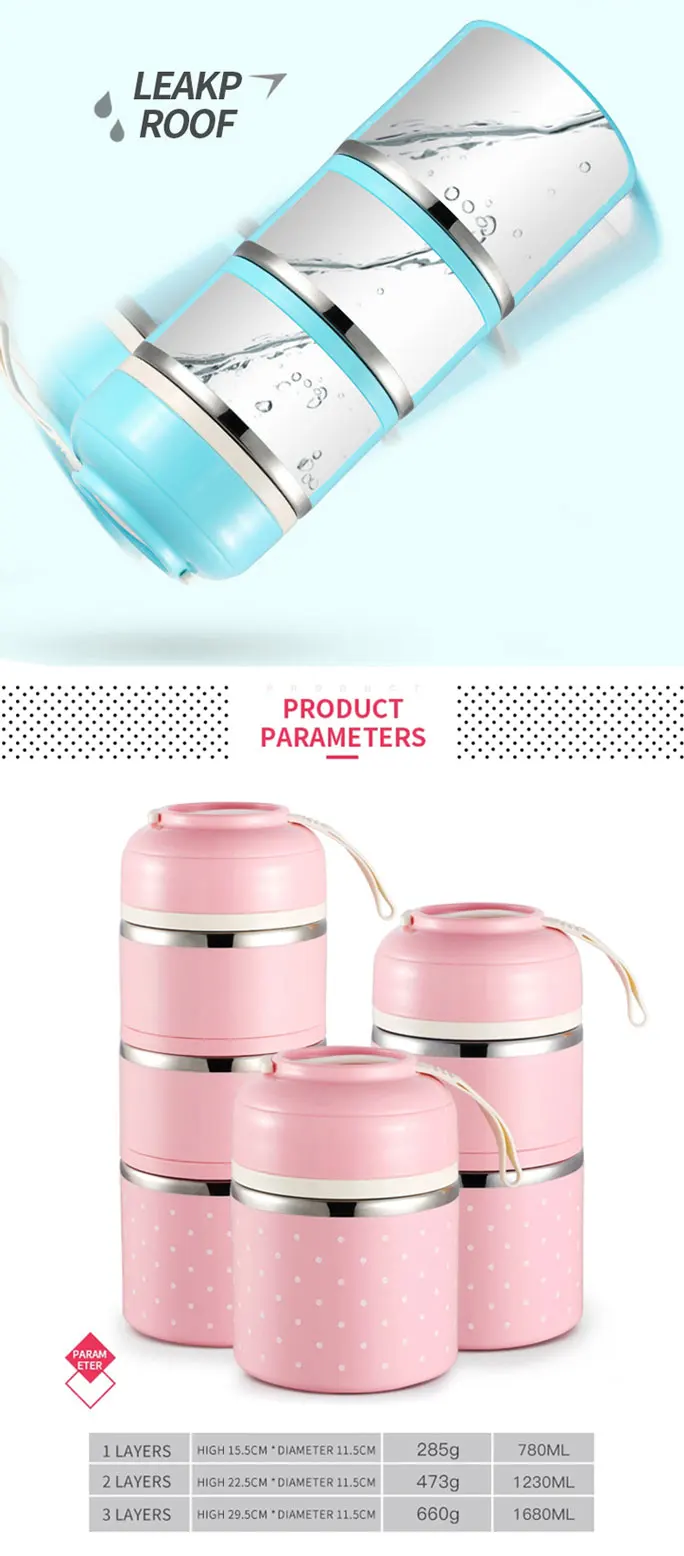
Usually, these tools categorize the keywords as genes/proteins, diseases, species, cell lines, and mutations. Given a text, a NER tool will categorize, for example, the words ’breast cancer’, ‘fever’, ‘migraine’ or ‘hepatites’ as diseases and ‘human’, ‘rat’, ‘patient’ or ‘person’ as species. Then, all remaining unique tokens are processed through different methods to extract features that represent biomedical classes to be transformed into a suitable representation. NER tools implement the text pre-processing stage, where all data are cleaned and tokenized, a step in which typically words are broken down into words or sub-word tokens to build the vocabulary of such text. Hence, this step identifies concepts and keywords, categorizing them in user-defined classes. Biomedical NER aims to retrieve relevant biomedical entities such as genes, drugs, diseases, species, proteins, mutations, and cell lines existent in natural language documents and tag each word´s location and class. NER, also known as ‘entity tagging’ or ‘concept extraction’, is fundamental for automatically extracting information from text.

Text mining comes upon the first step of automated information retrieval (IR) to retrieve all the information relevant to a specific problem from disperse data resources. BioNLP, the application of NLP models in the biomedical field, adds the required knowledge of specific biological contexts. An NLP model should understand the language, semantics, and vocabulary to predict token features correctly. Text mining applies NLP methods to extract and retrieve information from text just like a human reader would.

Text mining provides a set of automated methods that can distill text from heterogeneous sources into actionable data. Using text mining in the biomedical field has widely increased due to the emergent need to analyze and gain knowledge from large data sources. Often, these data are unstructured and not ready for computational interpretation. The amount of data available from scientific papers, patents, or other sources of information, particularly in the biomedical field, is continuously rising. In the pharmaceutical sector, automatic terminology extraction from pharmaceutical documents of meaningful information has been applied to classify documents.
BENTO IMPORT IDATABASE PROFESSIONAL
These were used to developed a specific terminology list of patients whilst browsing phrases to compare professional and common terminology and evaluate statistics of different terminologies in two different languages. In the health sector, particularly in diabetes, corpus-based terminology from online texts, manuals, or professional papers have been automatically extracted. Big text data in this sector, from websites or even social media, has been used on stock price prediction, financial fraud detection, and market forecast. Moreover, it has been increasingly applied in various industries, such as the financial sector for decision-making processes. Text mining is already widely used, mainly on social media, for, e.g., Twitter, to explore disease symptoms, reactions to public regulations, or to study the opioid crisis.
